Revolutionizing AI: Researchers Eliminate Matrix Multiplication in Language Models
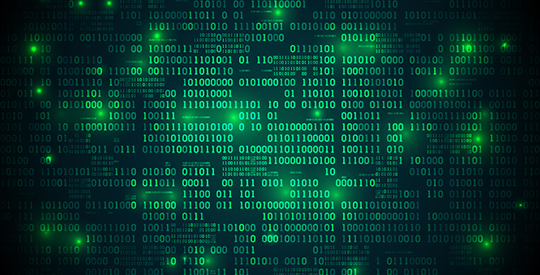
In a groundbreaking study, a team of researchers from the University of California Santa Cruz, UC Davis, LuxiTech, and Soochow University has redefined the operational framework of AI language models by eliminating the need for matrix multiplication, a staple in neural network computations. This innovation could dramatically reduce the environmental impact and operational costs associated with AI technologies.
Traditionally, matrix multiplication, or MatMul, is a core process accelerated by GPUs, crucial for the efficiency of neural networks. This dependency on GPUs, particularly highlighted by Nvidia’s dominant market share, underscores the significance of this research. The team’s work, detailed in their paper “Scalable MatMul-free Language Modeling,” introduces a custom 2.7 billion parameter model that operates without MatMul, maintaining performance levels comparable to conventional large language models (LLMs).
The researchers also tested a 1.3 billion parameter model on a custom-programmed FPGA chip, achieving efficient processing speeds at significantly lower power consumption—about 13 watts, excluding the GPU’s draw. This stark reduction from the conventional 700 watts for a similar GPU model indicates a potential 38-fold decrease in power usage, a substantial step toward sustainable AI development.
This MatMul-free approach not only challenges the traditional reliance on matrix operations for high-performing LMs but also opens the door to more accessible and sustainable AI deployments, particularly on devices with limited hardware capabilities like smartphones. The innovation extends beyond just energy savings; it significantly reduces memory usage during training, enhancing the feasibility of deploying advanced AI models in resource-constrained environments.
By replacing complex matrix operations with simpler arithmetic through innovations like the MatMul-free Linear Gated Recurrent Unit (MLGRU) and adapting Gated Linear Units (GLU) to ternary weights, the researchers have demonstrated that high efficiency and reduced computational demand do not have to compromise performance.
Their findings, while still pending peer review, suggest potential scalability that could rival, if not exceed, the capabilities of today’s leading LLMs at much larger scales. This breakthrough poses a promising horizon for AI technology, paving the way for more efficient, economical, and environmentally friendly AI operations globally.